Dani Chu is a second year masters student in statistics at Simon Fraser University. Recently, he completed an internship at the NBA department of basketball strategy and analytics. At SFU, he's the co-president of the SFU Sports Analytics Club with Lucas Wu and Matthew Reyers. Along with Lucas, Matt and James Thomson, he was the winner of the College Division of the 2019 NFL Big Data Bowl and the 2018 Sacramento Kings Case Competition. Dani has also interned as a statistician at Best Buy Canada and Fraser Health Authority.

About The Conference
Now in its third year, the Carnegie Mellon Sports Analytics Conference is dedicated to highlighting the latest sports research from the statistics and data science community.
Interested in presenting your research at CMSAC? Submit an abstract using the form below! And if you are using publicly available data then consider entering our second annual Reproducible Research Competition!
Stay tuned for more information about the upcoming #CMSAC19. Check out our 2017 and 2018 conferences.
Registration is Sold-out!
Early Bird Registration (until Oct 15th)
- High School students – FREE (with school ID)
- Undergrad/Grad students Conference: $20 (with school ID)
- Undergrad/Grad students Workshop: $10 (with school ID)
- Undergrad/Grad students Conference + Workshop: $25 (with school ID)
- Non-students Conference: $50
- Non-students Workshop: $20
- Non-students Conference + Workshop: $60
Regular Registration (Oct 16th - Nov 1st)
- High School students – FREE (with school ID)
- Undergrad/Grad students Conference: $25 (with school ID)
- Undergrad/Grad students Workshop: $10 (with school ID)
- Undergrad/Grad students Conference + Workshop: $30 (with school ID)
- Non-students Conference: $75
- Non-students Workshop: $20
- Non-students Conference + Workshop: $85
Registering indicates agreement to abide by the Code of Conduct .
Hotel information
We have a room block with The Oaklander Hotel (reserve now with this link), or make reservations by calling 877-829-2429 and ask for the CMU Sports Analysis Block.Conference Location
Carnegie Mellon University
Porter Hall 100
4909 Frew St, Pittsburgh, PA 15213
From PIT Airport
1. Head northeast on Airport Blvd
2. Keep left to stay on Airport Blvd - 0.6 mi
3. Keep left to stay on Airport Blvd - 0.7 mi
4. Continue straight to stay on Airport Blvd - 0.2 mi
5. Keep left at the fork, follow signs for
I-376 E/I-79 E/Pittsburgh/Pennsylvania Turnpike E and
merge onto I-376 E - 0.6 mi
6. Merge onto I-376 E - 16.4 mi
7. Keep right to stay on I-376 E - 2.1 mi
8. Take exit 72A to merge onto Forbes Ave toward Oakland - 0.3 mi
9. Merge onto Forbes Ave - 1.0 mi
10. Turn right onto Schenley Drive Extension - 449 ft
11. Turn left onto Schenley Drive - 0.2 mi
12. Turn left onto Frew St 0.2 mi
13. Destination will be on the left
Schedule Details
Football Analytics Workshop in Baker Hall A51, Giant Eagle Auditorium
Led by Ron Yurko, the CMSAC football analytics workshop is a three-hour event (5 to 8 PM), with the first hour dedicated to introducing attendees to reading, wrangling, and visualizing publicly available NFL data with the R statistical programming language, specifically using the tidyverse. The third hour of the workshop will cover the basics of using R to generate ELO ratings for NFL teams, a popular rating system featured on websites such as FiveThirtyEight. The middle hour of the workshop features our keynote speaker, Michael Lopez, who will discuss his work as the NFL Director of Data and Analytics. No prior programming experience is required, more information will be available soon.
-
5 PM
Into the tidyverse with NFL data
By Ron Yurko -
6 PM
Keynote Speaker: Michael Lopez
Director of Football Data and Analytics, NFL> -
7 PM
Introduction to NFL ELO ratings
By Ron Yurko
Conference sessions in Porter Hall 100
-
8:00 AM
Registration
-
8:50 AM
Welcome and Opening Remarks
Rebecca Nugent and Carnegie Mellon Sports Analytics Club> -
9:00 AM
Keynote Address: Cade Massey
The Wharton School of the University of Pennsylvania> -
9:45 AM
Coffee Break
-
10:00 AM
Scouting and Scoring: How We Know What We Know About Baseball
Christopher Phillips> -
10:25 AM
The 2019 Home Run Surge: A Whole New Ballgame (Again)
Meredith Wills> -
10:55 AM
Coffee Break
-
11:05 AM
Expected Hypothetical Completion Probability: An Analysis from the 2019 NFL Big Data Bowl
Sameer Deshpande> -
11:30 AM
Estimating Player Value in Football Using Plus-Minus Models
Paul Sabin> -
11:55 AM
CMSACamp Spotlight
Rebecca Nugent> -
12:05 PM
Poster Previews
Poster Presenters> -
12:15 PM
Lunch and Poster Session
-
1:30 PM
Vamos! Estimating Shot Value In Tennis with a Functional Spatiotemporal Gaussian Mixture Model
Stephanie Kovalchick> -
1:55 PM
From Grapes and Prunes to Apples and Apples: Using Matched Methods to Estimate Optimal Zone Entry Decision-Making in the National Hockey League
Asmae Toumi> -
2:20 PM
Growth Curves for Predicting Athlete Ratings
Katy McKeough> -
2:45 PM
Break
-
3:00 PM
A Bayesian hierarchical regression-based metric for NBA players
Brian MacDonald> -
3:25 PM
Running out of time: A hierarchical model for estimating foul trouble in the NBA
Dani Chu> -
3:50 PM
The causal effect of a timeout at stopping an opposing run in the NBA
Connor Gibbs> -
4:15 PM
Break
-
4:25 PM
Reproducible Research Competition Final Four Presenters and Awards
-
6:00 PM
Closing Remarks
Rebecca Nugent> -
6-7 PM
Networking Reception
Conference Keynote Speaker

Biography:
Cade Massey is a Practice Professor in the Wharton School’s Operations, Information and Decisions Department. He received his PhD from the University of Chicago and taught at Duke University and Yale University before moving to Penn in 2012. Massey’s research focuses on judgment under uncertainty – how well people predict what will happen in the future – and especially processes that blend experts and algorithms. His work draws on experimental and “real world” data such as employee stock options, 401k savings, the National Football League draft, and graduate school admissions. His research has led to long-time collaborations with Google, Merck and multiple professional sports franchises. Massey is faculty co-director of Wharton People Analytics, co-host of “Wharton Moneyball” on SiriusXM Business Radio, and co-creator of the Massey-Peabody NFL Power Rankings for the Wall Street Journal and Washington Post.
Workshop Keynote Speaker

Biography:
Mike Lopez is the Director of Football Data and Analytics with the NFL, and an adjunct professor and research associate at Skidmore College. He received his PhD in Biostatistics from Brown University in 2010. His research spans causal inference – with a specific focus on causal inference methods for multiple exposures or multiple exposure doses – and the application of statistics to sports.
Conference Speakers

Running out of time: A hierarchical model for estimating foul trouble in the NBA
NBA coaches are tasked with managing the playing time for each of their players. Throughout the season they develop lineups and rotations to give players the rest they need while maximizing the team’s chance at winning. But how should coaches adapt when a player is in foul trouble? Threatening the established game plan. Moreover, how do we quantify when a player is at a risk to not play their usual minutes? Typically, coaches use the Q+1 rule that says that a player is at risk of fouling out of a game when they have 1 more foul than the current quarter. This generic foul trouble calculation treats all players equally, despite many players having known tendencies with respect to foul acquisition. Our objective is to outline player personalized guidelines for a coaching staff to handle in-game foul management. To do so we fit a Hierarchical Bayesian model with NBA play by play data to estimate the distribution of time to foul out for a given player with a given number of fouls. We build this into a framework that can later incorporate factors such as fatigue, defensive responsibilities, restrained performance and game situation. We find that this personalized and data driven method to understanding when a player will foul out is more useful than a catchall approach and lays the groundwork to a more nuanced discussion of player rotations.

Sameer is a post-doctoral researcher at MITs Computer Science and Artificial Intelligence Laboratory. He received his Ph.D. in Statistics from the Wharton School at the University of Pennsylvania in 2018. His methodological research primarily focuses on Bayesian model selection, clustering, and model averaging. In addition to studying the effects of playing football, he has previously worked on estimating how NBA players help their teams win games and on quantifying the uncertainty about the value of pitch framing in baseball. Outside of statistics, he is an avid sports fan, with particular affection for Dallas-based teams.
Expected Hypothetical Completion Probability: An Analysis from the 2019 NFL Big Data Bowl
Using high-resolution player tracking data made available by the National Football League (NFL) for their 2019 Big Data Bowl competition, we introduce the Expected Hypothetical Completion Probability (EHCP), a objective framework for evaluating plays. At the heart of EHCP is the question 'on a given passing play, did the quarterback throw the pass to the receiver who was most likely to catch it?' To answer this question, we first built a Bayesian non-parametric catch probability model that automatically accounts for complex interactions between inputs like the receiver's speed and distances to the ball and nearest defender. While building such a model is, in principle, straightforward, using it to reason about a hypothetical pass is challenging because many of the model inputs corresponding to a hypothetical are necessarily unobserved. To wit, it is impossible to observe how close an un-targeted receiver would be to his nearest defender had the pass been thrown to him instead of the receiver who was actually targeted. To overcome this fundamental difficulty, we propose imputing the unobservable inputs and averaging our model predictions across these imputations to derive EHCP. In this way, EHCP can track how the completion probability evolves for each receiver over the course of a play in a way that accounts for the uncertainty about missing.

Connor Gibbs is a second year PhD student in the department of Statistics at Colorado State University. He graduated summa cum laude from the University of Georgia in May of 2018 with a B.S. in Mathematics and a B.S. in Statistics. Apart from his work in causal inference, Connor enjoys hiking and traveling.
The causal effect of a timeout at stopping an opposing run in the NBA
In the summer of 2017, the NBA reduced the total number of timeouts, among other rule changes, to regulate the flow of the game. With these rule changes, it becomes increasingly important for coaches to effectively manage their timeouts. Understanding the utility of a timeout under various game scenarios, e.g. during an opposing team’s run, is of the utmost importance. There are two schools of thought when the opposition is on a run: (1) call a timeout and allow your team to rest and regroup, or (2) save a timeout and hope your team can make corrections on the fly. This talk investigates the credence of these tenets using the Rubin causal model framework to quantify the causal effect of a timeout in the presence of an opposing team’s run and provides coaches with the needed analytic justification for better in-game decision making.

Stephanie Kovalchik
Tennis Australia and Institute for Health and Sport at Victoria University in Melbourne
Dr. Stephanie Kovalchik is a senior data scientist in the Game Insight Group at Tennis Australia and is currently a Research Fellow at the Institute for Health and Sport at Victoria University in Melbourne, Australia. Dr. Kovalchik earned her PhD and MS in Biostatistics from UCLA and her BS in Biology from Caltech. Her current research focuses on the use of statistical methods to understand performance, game strategy, and mentality in high-performance tennis.
Vamos! Estimating Shot Value In Tennis with a Functional Spatiotemporal Gaussian Mixture Model
Every point in tennis is a battle for control over the space and time of a tennis ball. Despite this, performance statistics in tennis largely ignore the spatio-temporal features of points. Taking inspiration from several high-resolution models that have been developed to estimate the value of actions in professional team sports, the present work develops a framework for modeling the space-time evolution of shots that allows us to estimate expected shot value (ESV) continuously throughout a tennis point. Key to our approach is the encoding of shot and player trajectories into a lower-dimensional representation that retains all available space-time information. An infinite Bayesian Gaussian mixture model provides a generative distribution for the 3D ball and 2D player trajectories that is in good agreement with real tracking data. In this talk, I will describe this fundamental building block of the ESV approach and show how ESV can be used to gain deeper insights into player skill and decision-making.

Brian is currently the Director of Sports Analytics in the Stats & Information Group at ESPN. He was previously the Director of Hockey Analytics with the Florida Panthers Hockey Club, an Associate Professor in the Department of Mathematical Sciences at West Point, an Adjunct Professor in the Department of Management Science at the University of Miami, and an Adjunct Professor in Sports Analytics in the College of Business at Florida Atlantic University. He received a Bachelor of Science in Electrical Engineering from Lafayette College, Easton, PA, and a Master of Arts and a Ph.D. in Mathematics from Johns Hopkins University, Baltimore, MD.
A Bayesian hierarchical regression-based metric for NBA players
We present a Bayesian hierarchical regression model that estimates the value of box score statistics and player coefficients simultaneously, and provides an estimate of an NBA player’s contribution to his team’s on-court performance. We discuss how our approach differs from other regression-based metrics, provide visualizations of those differences over time as a way to highlight the characteristics of each, and discuss how this approach could be used in hockey, soccer, football, or eSports.

Katy McKeough
Harvard University Statistics Department
Katy McKeough is a fifth-year Ph.D. student in the Harvard University Statistics Department. Her research involves using advanced statistical models in applied settings including sports analytics and astrostatistics. She graduated from Carnegie Mellon University in 2015 with a degree in physics with a secondary major of statistics. She is a member of both the CHASC: Astrostatistics Group and the Sports Analytics Lab at Harvard.
Growth Curves for Predicting Athlete Ratings
It is often the goal of sports analysts, coaches and fans to predict athlete performance over time. Methods such as Elo, Glicko and Placket-Luce based ratings measure athlete skill based on results of competitions over time but have limited predictive strength on their own. Growth curves are often applied in the context of sports to predict future ability, but these curves are too simple to account for complex career trajectories. We propose a mixture of non-linear, mixed-effects growth curves to model the ratings as a function of athlete age and time. The mixture of growth curves allow for flexibility of the estimated shape of the career trajectories between athletes as well as between sports. We use the fitted growth curves to make predictions about the future career trajectory of an athlete. We apply this method to men's slalom results but it can be generalized to other sports.

Christopher J. Phillips is Associate Professor of History at Carnegie Mellon University. He received his PhD in History of Science from Harvard University and also taught at New York University before coming to CMU in 2015. Phillips’s research focuses on the history of statistics, and in particular, the supposed benefits of introducing numbers and analytics into new fields. He is the author of Scouting and Scoring: How We Know What We Know About Baseball (Princeton University Press) and also serves as an Associate Editor for the Harvard Data Science Review.
Scouting and Scoring: How We Know What We Know About Baseball
Amateur scouting and data-driven analytics are presumed to be two very different ways of measuring quality and success in baseball, one focused on qualitative and subjective evaluation of prospects’ bodies, the other on quantitative and objective evaluation of playing statistics. The two camps—scouts and scorers—are sometimes portrayed as opposed or irreconcilable. Historically, however, their practices were far more similar than different. Both relied on numbers, bureaucratic management, and human labor to make reliable judgments. By unearthing the history of official scorers and database creators, scouting reports and bureaus, we can come to understand a new history of data analytics and scouting in baseball.

Paul Sabin is a Senior Sports Analytics Specialist at ESPN. He received his PhD in statistics from Virginia Tech after receiving an MS in Statistics and a BS in statistics and French at BYU. Paul has built several publically facing ESPN metrics including the suite of CBB metrics (BPI, SOR), NBA Draft Prospect Projections, Soccer fantasy projections, the Allstate Playoff Predictor (CFB), and the Playstation Player Impact Rating (CFB). He is also a contributor to ESPN.com. Outside of ESPN, Paul researches hierarchical, multiscale, and Dirichlet Bayesian methods and TV ratings in sports. Paul is forever a hopeless BYU and DC sports fan plus a hopeful fan of all teams featuring Kylian Mbappe, whose play converted him to soccer fandom.
Estimating Player Value in Football Using Plus-Minus Models
To date calculating the value of football players on-field performance has been limited to scouting methods and quarterbacks. A popular method to calculate player value in other sports are Adjusted Plus-Minus (APM) models. These models have long been used in other sports, most notably basketball (Rosenbaum (2004), Kubatko et al. (2007), Winston (2009), Sill (2010)) to estimate each players value by accounting for those in the game at the same time. Football is the least amenable major American sport to APM models due to its few scoring events, few lineup changes, restrictive positioning, and small quantity of games relative to the number of teams. More recent methods have found ways to incorporate plus-minus models in sports such as Hockey (Macdonald (2011)) and Soccer (Schultze and Wellbrock (2018) and Matano et al. (2018)). These models are especially useful in coming up with results-oriented estimation of each player’s value. In American Football, it is difficult to estimate every player’s value since many positions such as offensive lineman have no recorded statistics. While player-tracking data in the NFL is allowing new analysis, such data does not exist in other levels of football such as the NCAA. Using player participation data available for college football and the NFL, I provide a model framework that solves many of the traditional issues APM models face in football. Results for these APM models are provided for both Collegiate and NFL football players. Additionally, this methodology allows the models to estimate the value of each position in each level of the sport.

As editor-in-chief of Hockey-Graphs, Asmae manages the day-to-day operations and oversees the editorial and creative process. In her role, she spearheaded a mentorship program pairing NHL data scientists and executives with underrepresented persons. She has hosted multiple workshops in data viz and modelling at MIT Sloan Sports Analytics Conferences. She also works for the Massachusetts General Hospital Institue of Technological Assessment as a Data Analyst.
FROM GRAPES AND PRUNES TO APPLES AND APPLES: USING MATCHED METHODS TO ESTIMATE OPTIMAL ZONE ENTRY DECISION-MAKING IN THE NATIONAL HOCKEY LEAGUE
Previous research in the National Hockey League has suggested that teams' decisions to gain the offensive zone with puck possession ("carry-ins") is preferred over dumping the puck in and chasing after it ("dump-ins"). However, standard comparisons of zone entry strategy are confounded by factors such as offensive and defensive talent, location on the ice, and shift time, each of which impact player choice. Indeed, contrasting carry-ins to dump-ins isn’t exactly an apples-to-apples comparison; instead, it is more like studying grapes versus prunes. Using two matching methods – propensity score matching and Bayesian additive regression trees – we leverage player-tracking data to estimate the causal benefits due to zone-entry decisions. Both approaches better account for the variables that affect entry choice. We also highlight the wide-ranging potential of the causal inference framework with player tracking data in sports while emphasizing the challenges of using standard statistical methods to inform decision-making in the presence of substantial confounding.

Meredith J. Wills, Ph.D., is a Data Scientist for SportsMEDIA Technology (SMT). She has a B.A. in Astronomy & Astrophysics from Harvard University, and an M.S. and Ph.D. in Physics from Montana State University—Bozeman. Dr. Wills joined SMT in 2018, where she works primarily with FIELDf/x, a baseball ball- and player-tracking system used by Minor League affiliates of Major League ballclubs, international leagues, and NCAA. She also writes for The Athletic, and her best-known independent research involves MLB baseball construction and its effect on the game.
The 2019 Home Run Surge: A Whole New Ballgame (Again)
In 2017, Major League Baseball saw an unprecedented increase in home runs. It was determined that this Home Run Surge was caused by a physical change to the ball. By disassembling a sample of baseballs and studying their construction, I found that the introduction of thicker laces ultimately produced a more aerodynamic ball. This past season, MLB’s home run rate soared even farther, and was once again related to changes in baseball construction. Using similar methods, I disassembled a sample of 2019 baseballs and compared their properties to those of earlier populations. This time, my findings showed that multiple aspects of the ball had changed, and that these differences could account for lower drag and a higher home run rate. Evidence suggests that the changes were due to manufacturing process modifications and better quality control, and that the extent of the ball’s aerodynamic improvement—while perhaps not unwelcome—was likely unexpected.
Reproducible Research Competition Finalists
(alphabetical order by first author)



Heejong Bong, Wanshan Li, Shamindra Shrotriya (view paper)
Department of Statistics & Data Science, Carnegie Mellon University
Efficient Estimation of Distribution-free dynamics in the Bradley-Terry Model
We propose a time-varying generalization of the original Bradley-Terry model. Our model directly captures the temporal dependence structure of the pairwise comparison data to model time-varying global rankings of N distinct objects. The convex formulation enables efficient analysis on sparse time-varying pairwise comparison data. Furthermore, depending on the choice of penalization norm, our model effectively provides a control on the degree of smoothing in the time-varying global rankings. We also prove that a relatively weak condition is necessary and sufficient to guarantee the existence and uniqueness of the solution of our model. Our condition is the weakest in literature till now. We implement various optimization algorithms to solve the model efficiently. We test the practical effectiveness of our model by separately ranking 5 seasons of publicly available National Football League (NFL) team data from 2011-2015, and NASCAR 2002 racing data. In particular, our ranking results on the NFL data compare favourably to the well-accepted and feature-rich NFL ELO ratings system. We thus view our time-varying Bradley-Terry model as a useful benchmarking tool for other feature-rich time-varying ranking models since it simply relies on the minimal time-varying pairwise comparison results for modeling.
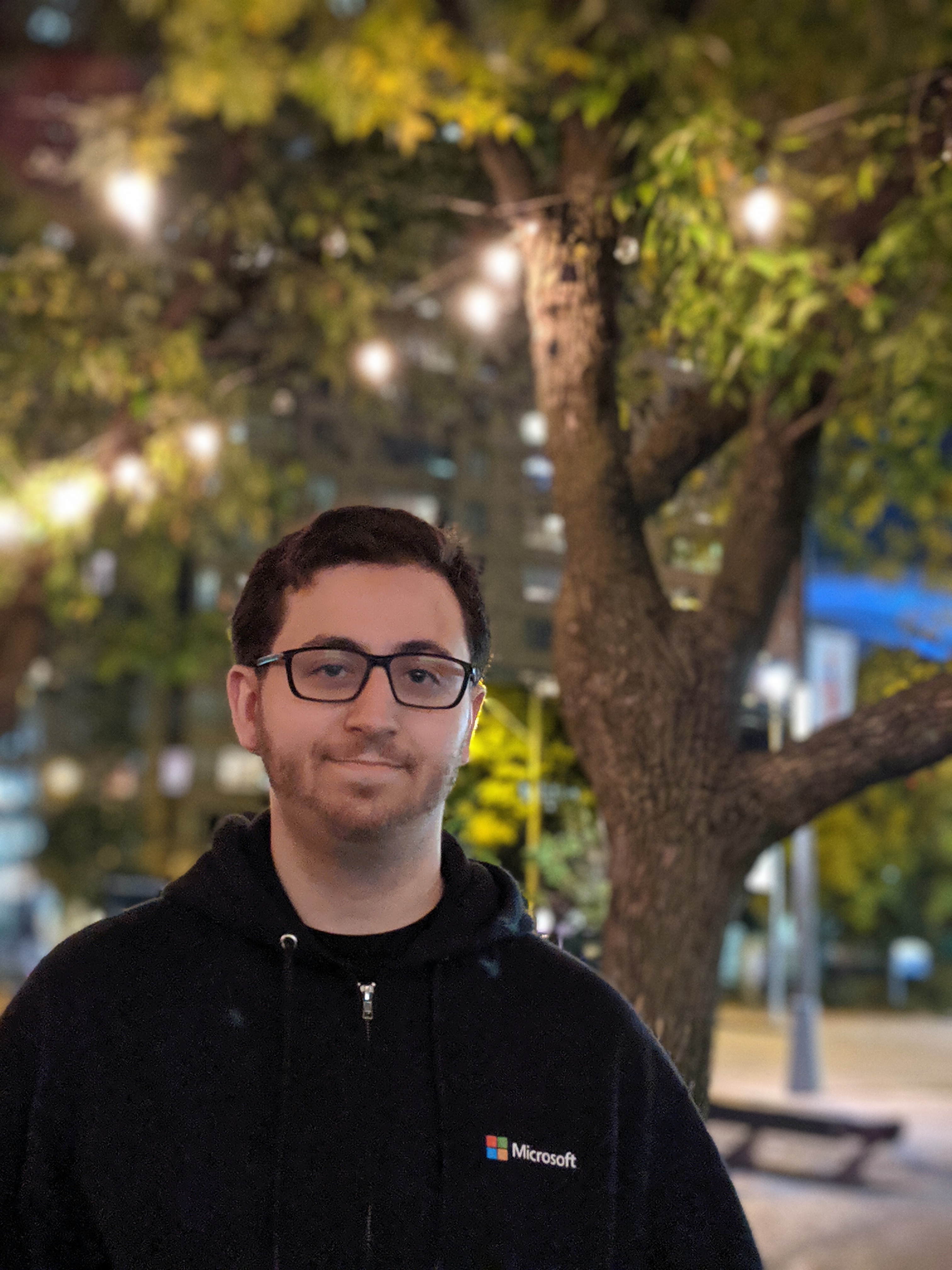
Jacob Danovitch (view paper)
Carleton University
Trouble with the Curve: Predicting Future MLB Players Using Scouting Reports
In baseball, a scouting report profiles a player’s characteristics and traits, usually intended for use in player valuation. This work presents a first-of-its-kind dataset of almost 10,000 scouting reports for minor league, international, and draft prospects. Compiled from articles posted to MLB.com and Fangraphs.com, each report consists of a written description of the player, numerical grades for several skills, and unique IDs to reference their profiles on popular resources like MLB.com, FanGraphs, and Baseball-Reference. With this dataset, we employ several deep neural networks to predict if minor league players will make the MLB given their scouting report. We open-source this data to share with the community, and present a web application demonstrating language variations in the reports of successful and unsuccessful prospects.

Jacob Richey (view paper)
The Wharton School of the University of Pennsylvania
playerElo
With the sabermetric revolution of the MLB, a plethora of new statistics have come into the mainstream, and a growing number of fantasy owners, ballclubs, and regular fans are turning to these new statistical methods for player analysis. However, I propose even advanced metrics such as wOBA, FIP, xwOBA, xFIP, and wRC+ are all missing a crucial element to accurately represent player performance thus-far. The playerElo system is able to reveal in aggregate the effects of previously unconsidered aspects of the game. Using an Elo ranking system determined by run-value calculations of all major league baseball players, the model incorporates context-dependent analysis and quality of competition to produce a proper evaluation of batters and pitchers. This enables playerElo to appropriately credit pitchers, especially relievers, for their true impact on the game, particularly when called upon in disadvantageous situations. Additionally, playerElo does not allow relative team strength, which confounds common counting statistics, to influence the evaluation of a player. The model is a holistic approach to the assessment of major league players and has incredible ramifications on player projections during free agency and player acquisition.
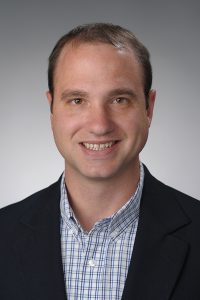

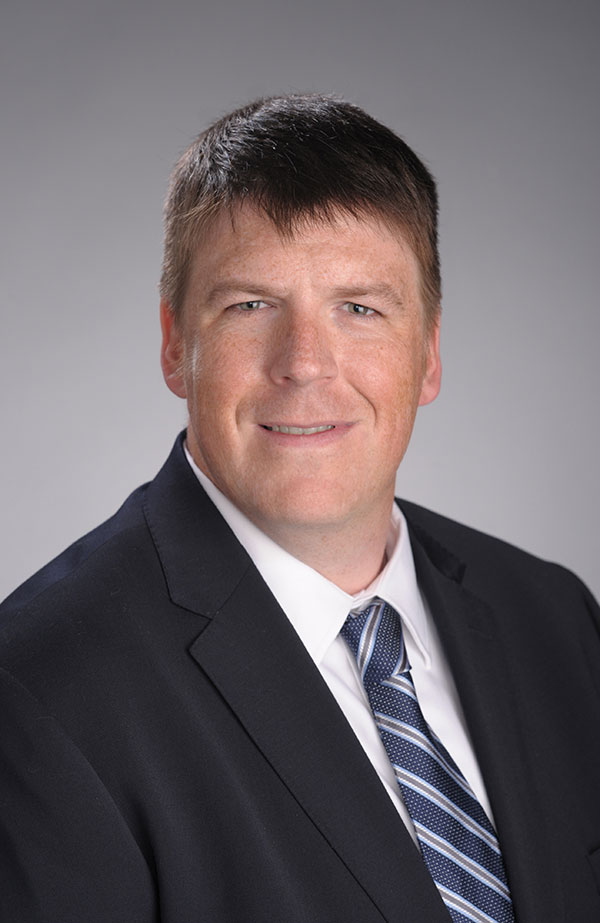
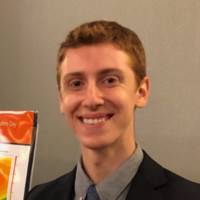
Shane Sanders1, Joel Potter2, Justin Ehrlich1, Justin Perline1 (view paper)
1Syracuse University, 2 University of North Georgia,
Wins Above Replacement and the MLB MVP Vote: A Natural Experiment
Wins above replacement is an objective measure of player on-field value in MLB. The measure was created in 2004 and subsequently popularized on leading MLB data sites such as Baseball Prospectus, Baseball Reference, ESPN and Fangraphs. The creation of WAR provides a natural experimental setting. Before 2004, Baseball Writers of America members cast MLB MVP ballots absent a comprehensive player (win contribution) value measure. This was a daunting task given the apples-to-oranges nature of cross-positional comparisons in baseball. Although WAR was not available to MVP voters before 2004, it was retroactively calculated throughout MLB history using data from sources such as retrosheet.org (such that sabermetricians and baseball historians can and do fuel their GOAT arguments with actual player value measurement). We use these retroactive calculations to estimate the relationship between WAR-estimated player value and MVP voting before 2004. We also estimate this relationship for votes that occurred from 2004 through 2017. Across sets of both fixed effects negative binomial and neural network regression models, we find significant and substantial evidence that the effect of WAR upon MVP vote points was significantly and substantially stronger. from 2004-2017 than from 1980-2003. A unit of additional WAR was worth an additional 37 (45) vote points, in expectation, from 1980-2003 (2004-2017). Further, we present evidence that this shift in voting behavior was not a gradual response to the sabermetric era in general but rather a specific response to the creation of WAR. Namely, voting behavior from 1992-2003 was almost identical to that from 1980-1991. Following the advent of WAR, informed voters were more likely to select the most qualified players.